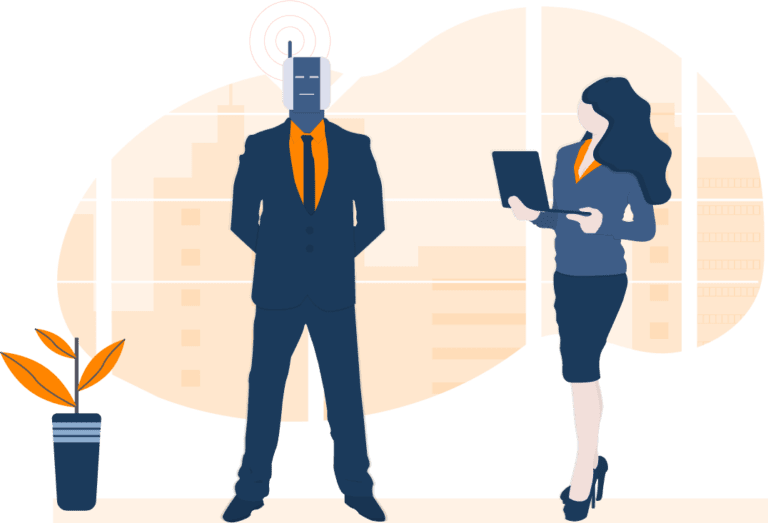
The weak maturity of data governance projects necessitate the implementation of good practices and feedback loops to constantly monitor and verify the validity of management rules on your data asset.
The following articles explain the characteristics of a data governance labeled as agile in order to:
1. Be as close as possible to your enterprise’s operational reality.
2. Adapt to your enterprise’s context and not the other way around.
3. Accurately reflect your data assets.
4. Unify and involve your collaborators.
5. Respond to changes quickly.
Implementing data governance must begin by referencing, indexing, and evaluating your organization’s data assets. Building such an artifact by only using human intelligence is rarely successful given the resource constraints. Therefore, it is necessary to maximise automated processes regarding the extracting and collecting of information related to the data in your organization.
With the help of artificial intelligence algorithms or machine learning, it becomes possible to interpret, contextualize, and give more meaning to your data assets.
With the help of artificial intelligence algorithms or machine learning, it becomes possible to interpret, to contextualize, and to deduce a more precise meaning to your data asset. This automation allows your data managers avoid the blank paper syndrome and, freeing them from tedious and repetitive tasks, increase the support from as many people as possible of your initiative to implement data governance within your company.
Finally, this automation became a necessity in the new era where the volume and the variety of systems exploded. The maintenance and the updating of this information repository are crucial to reflect the reality of your IS data. With the help of incremental analysis, which frequently and automatically processes your data, you will gain a high-value metadata repository added for your data consumers.